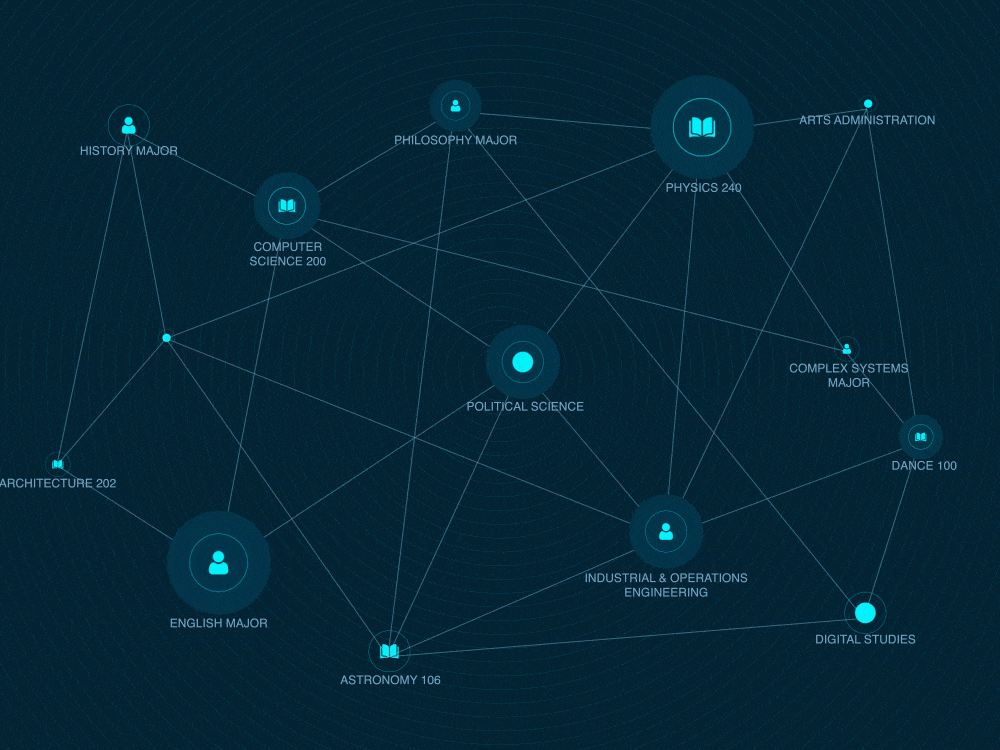
When you think about places at U-M that attract students from all over campus who might not otherwise overlap, you might think of the Diag, the cafeteria in MoJo, or the Big House. But alumnus Uriah Israel (Ph.D. ’20) says you should probably consider classrooms too.
“Astronomy 106, which is a course about aliens, and Dance 100 connect more people who wouldn’t normally take a class together more often than any other courses on campus. A liberal arts education is supposed to expose students to new ideas and new people,” he says. “We wanted to see which courses facilitate that experience.”
For his doctorate in applied physics and a graduate certificate from the Center for the Study of Complex Systems, Israel used big data techniques to analyze enrollment data to develop different ways to measure a student’s educational experience. “We looked at every course that every student took for the past 15 years. It’s a lot of data,” Israel says. “We viewed the data as one large network, or graph, and studied it all using network analysis.”
Representing the data as a network requires two components: nodes and edges. Nodes are the things in the network, and edges are their connections. In this network, courses and students play both roles. Two students can be connected to one another when they take the same course. Two courses can be connected to one another when the same student takes both.
Network theory can be used to study how the nodes in a network interact. Israel analyzed this network of students and courses to explore campus connections. “By laying out all the nodes—students and courses—and drawing edges between them, we could not only see how students are connected via the courses they take,” Israel says. “We could also see how courses are connected to other courses by students who are taking them.”
The common assumption is that every person who graduates with the same major enrolls in similar courses, but in the liberal arts, Israel found that was not necessarily true. “With majors such as industrial and operations engineering, one cohort of students pretty much takes the same courses, so their major is really representative of which courses they took,” Israel says.
“But when you look at an English major,” he continues, “everyone takes some core classes, but there is a lot of variation between one student’s course selections and the next.” Through elective courses, an English major’s coursework might end up looking more like the courses you’d take to get a political science degree. This is an important observation, Israel says, because it illustrates the principles behind a liberal arts education. “The liberal arts are designed on the belief that there are multiple ways to learn something, whether it’s through a writing course, a history course, or a philosophy course.”
Analysis of these networks, Israel explains, shows that a liberal arts student isn’t fully defined by their major. “But the cool thing is that by using data science and machine learning, you see which kinds of courses actually align with each other and can come up with new definitions and classifications systems.”
Israel wondered if there might be a different way to classify students’ education that captures how people with different core interests pursue similar studies. “It could be a way to better communicate the intentional design of the liberal arts—the freedom to not be bound to one track within a major—that’s beneficial for students after they graduate,” he says.
Same Theories, Different Applications
Universities across the country are now using Israel’s research to create a safe semester in the midst of COVID-19. Instead of illustrating all of the possible intersecting patterns, they’re using his models to minimize student contact. It’s an inverse application, but the computational framework and data analysis of his graduate research remains vital. “The people making those decisions are using the same kind of coding, but instead of saying that mixing between students across campus is a positive thing, they’re looking at what things can be done to the network, to the student population, to make it not as mixed.”
Given the relevancy of this work, it’s surprising that, before graduate school, Israel didn’t know anything about machine learning. “I thought I would just be strictly physics. But then I learned about complex systems and was like, wait, the computer does what?! I thought it was so cool.”
In May 2020, Israel started a postdoctoral fellowship at Caltech in the Departments of Biology and Bioengineering and Computational and Mathematical Sciences, researching how to apply the methods of machine learning to better understand biology. It might not look the same as analyzing student enrollment data, but by using computers to perform machine learning and applying those methods to discover new ways to classify phenomena in biological data, Israel is still relying on his complex system foundation: searching for unexpected connections.
“At first, we just look at the data to see if it aligns with our intuition,” Israel says. “But then we take it further to see what surprises we can find. That’s when it gets interesting.”
- Learn More About Supporting the Department of Physics
- Learn More About Supporting the Center for the Study of Complex Systems